Migrating your Home Assistant database from SQLite to PostgreSQL can significantly enhance performance, especially as your data grows. This guide will walk you through creating a database dump, converting data types, setting up your PostgreSQL database, and configuring Home Assistant to use the new database.
Creating the Database Dump from SQLite
To start, you need to create a dump of your existing SQLite database:
sqlite3 home-assistant_v2.db .dump > ha_dump.sql
This command creates a plain text file containing the SQL commands needed to reconstruct the database.
Converting Data Types
During the migration from SQLite to PostgreSQL, certain data types need to be converted:
- DATETIME to TIMESTAMP: PostgreSQL uses
TIMESTAMP
instead ofDATETIME
.sql
sed -i 's/DATETIME/TIMESTAMP/g' ha_dump.sql
- BLOB to BYTEA: Convert BLOB fields to BYTEA for binary data.
sed -i 's/BLOB/BYTEA/g' ha_dump.sql
Preparing PostgreSQL Database
Creating Database and User
Start by setting up your PostgreSQL database and user:
CREATE DATABASE homeassistant; CREATE USER ha WITH ENCRYPTED PASSWORD 'yourpassword'; GRANT ALL PRIVILEGES ON DATABASE homeassistant TO ha;
Create the objects and load the data
Use the command line utility psql
to create the database objects and load the data into the newly created PostgreSQL database:
psql -h [your_db_host]-U ha -d homeassitant -f ha_dump.sql -W > load.log 2>&1
Instructions:
- Replace
[your_db_host]
with the actual hostname or IP address where your PostgreSQL database is hosted. - User and Database: Ensure that
ha
is the correct username andhomeassistant
is the correct database name you created for Home Assistant.
After running the command, the SQL dump file (ha_dump.sql
) will be executed against your PostgreSQL database. The output and any errors encountered during the process will be redirected to load.log
. This log file is essential for tracking the progress and identifying any issues that need resolution.
Check for Errors:
- Review the
load.log
file for possible errors. This file contains all output from thepsql
command, including any SQL errors or warnings that were generated during the import process. - Iterate as Necessary: If errors are found, you may need to fix issues in
ha_dump.sql
and rerun the command. This step might need to be repeated several times. Modifications could involve correcting data types, adding missing sequences for auto-incrementing fields, or adjusting SQL syntax to be compatible with PostgreSQL.
This process can be time-consuming, but it is crucial for ensuring that your database is correctly set up with all the necessary data and schema configurations. As noted, your mileage may vary depending on the specifics of your data and the initial state of the ha_dump.sql
file.
Adjusting the Schema
For tables needing auto-increment functionality (which is common in primary key columns), set up sequences:
CREATE SEQUENCE states_state_id_seq;
ALTER TABLE states ALTER COLUMN state_id SET DEFAULT nextval('states_state_id_seq');
SELECT setval('states_state_id_seq', (SELECT MAX(state_id) FROM states) + 1);
Repeat this pattern for other necessary tables and columns, such as events(event_id)
, state_attributes(attributes_id)
, and so on.
Configuring Home Assistant
Install SQLAlchemy and other dependencies
Home Assistant uses SQLAlchemy as the SQL toolkit and Object-Relational Mapping(ORM)system for Python. Install it with pip:
pip3 install SQLAlchemy
pip3 install psycopg2-binary
Modify the configuration
Modify the configuration.yaml
file to point to the new PostgreSQL database:
recorder: db_url: postgresql://ha:yourpassword@localhost/homeassistant
This setup directs Home Assistant to use the newly configured PostgreSQL database.
Benefits of Migrating to PostgreSQL
Moving from SQLite to PostgreSQL offers several benefits:
- Scalability: PostgreSQL handles larger databases and more concurrent connections.
- Performance: Improved query performance and optimization options.
- Reliability: Robust transaction support and recovery features.
- Flexibility: Richer set of data types and full-text searching capabilities.
Conclusion
Migrating your Home Assistant database to PostgreSQL not only enhances performance but also provides a more robust and scalable backend, suitable for growing smart home environments. This migration ensures that your Home Assistant setup can handle increased data loads efficiently and reliably.
Famous last words
One of my primary concerns during this migration was the potential loss of historical data, particularly how it might affect critical metrics like energy usage. The statistics
table, which was the last to have the auto-incremental column added, is pivotal as it houses the energy usage stats.
As the image below shows, there appears to be a gap of approximately four hours in the data on energy usage stats. However, it seems that Home Assistant has effectively compensated for this missing data. The system appears to have aggregated the missing energy usage from those four hours into the data represented in the 5 PM bar on the chart.
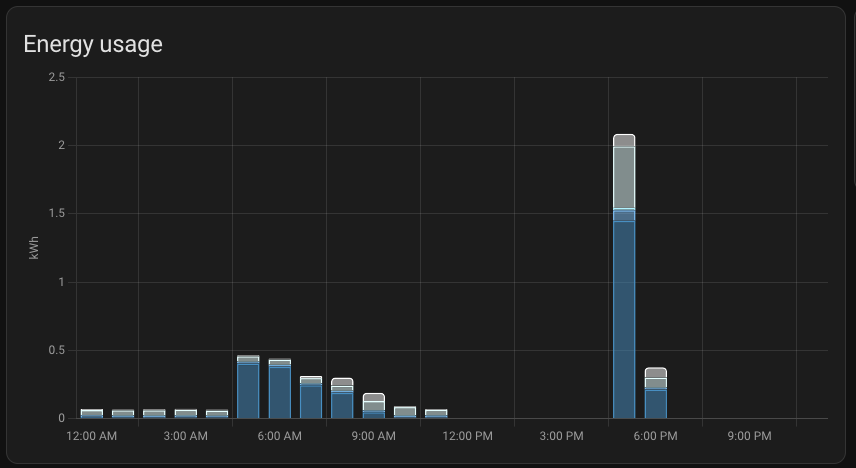
This outcome is quite reassuring and confirms that the system’s integrity remains intact despite the migration hiccups. I’m relieved to see that after all the adjustments and troubleshooting, everything is functioning as expected.
This experience underscores the importance of careful planning and execution in database migrations, especially when dealing with essential home automation systems like Home Assistant. The transition may require significant effort and attention to detail, but the end result can be gratifying, ensuring continuity and robustness in data handling.